
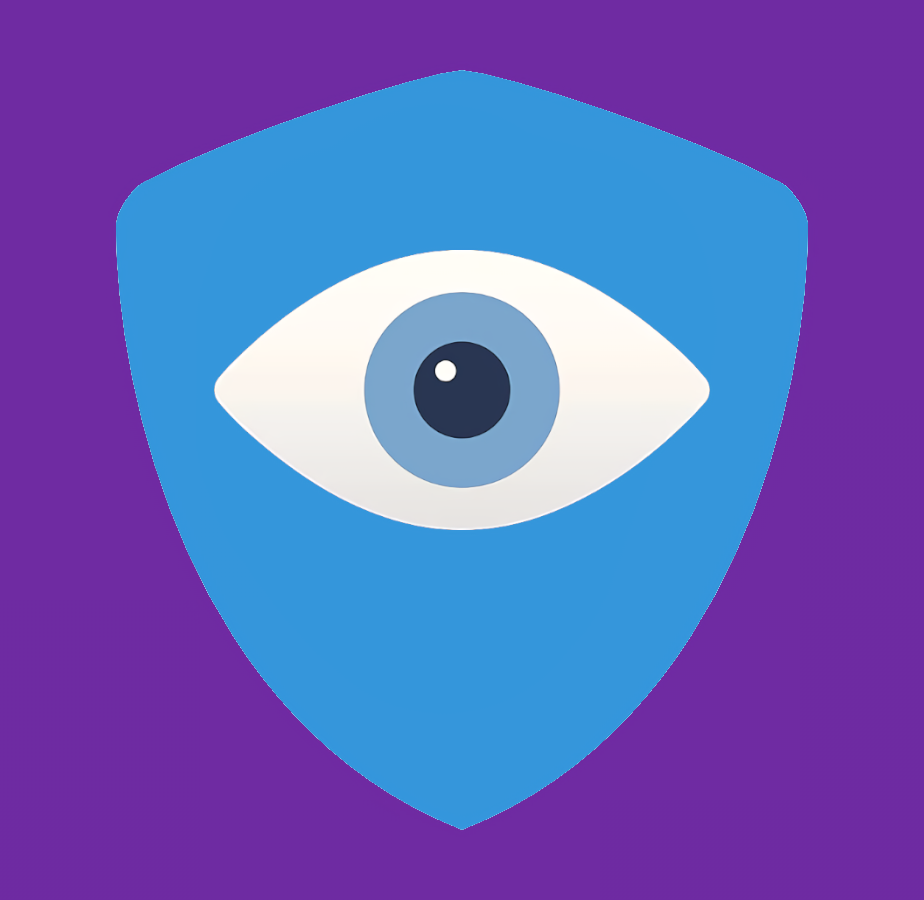
It renders fine, it’s just a pain to read due the wide aspect ratio. Either it’s too small or you have to scroll horizontal for reach line, or you have to flip your phone. None of it it is optimal.
It renders fine, it’s just a pain to read due the wide aspect ratio. Either it’s too small or you have to scroll horizontal for reach line, or you have to flip your phone. None of it it is optimal.
Well, well, well, now you think of the eggs. Real Women just have 'em free you know. /s
Fur real? I think you’re purrbably right.
Why do I really want to drink it? The distillation removes any radioactive particles making it safe to drink. And it just seems so fucking metal??
How many fingers? 🖐️
Fucking real player😩
TBF iTunes is a terrible player but made the shit loads of money so I guess they achieved what they set out to do.
And I would argue iTunes is the reason for newer media player versions being shit since of course MS saw that there was money to be made and tried to do the same.
What a bullshit argument. One of the arguments for self driving cars is precisely that they are not doing the same thing humans do. And why should they? It’s ludicrous for a company to train them on “social norms” rather than the actual laws of the road. At least when it comes to black and white issues as what is described in the article.
I don’t get the game tbh. At first it was cozy then it turned into work. Which I know people like, some at least. But I work enough in the day to not want to work when I play.
I’m not sure if you’re disagreeing with the essay or not? But in any case what you’re describing is in the same vein, that is simply repeating a word without knowing what it actually means in context is exactly what LLMs do. They can get pretty good at getting it right most of the times but without actually being able to learn the concept and context of ‘table’ they will never be able to use it correctly 100% of the time. Or even more importantly for AGI apply reason and critical thinking. Much like a child repeating a word without much clue what it actually means.
Just for fun, this is what Gemini has to say:
Here’s a breakdown of why this “parrot-like” behavior hinders true AI:
- Lack of Conceptual Grounding: LLMs excel at statistical associations. They learn to predict the next word in a sequence based on massive amounts of text data. However, this doesn’t translate to understanding the underlying meaning or implications of those words.
- Limited Generalization: A child learning “table” can apply that knowledge to various scenarios – a dining table, a coffee table, a work table. LLMs struggle to generalize, often getting tripped up by subtle shifts in context or nuanced language.
- Inability for Reasoning and Critical Thinking: True intelligence involves not just recognizing patterns but also applying logic, identifying cause and effect, and drawing inferences. LLMs, while impressive in their own right, fall short in these areas.
Interesting you focus on language. Because that’s exactly what LLMs cannot understand. There’s no LLM that actually has a concept of the meaning of words. Here’s an excellent essay illustrating my point.
The fundamental problem is that deep learning ignores a core finding of cognitive science: sophisticated use of language relies upon world models and abstract representations. Systems like LLMs, which train on text-only data and use statistical learning to predict words, cannot understand language for two key reasons: first, even with vast scale, their training and data do not have the required information; and second, LLMs lack the world-modeling and symbolic reasoning systems that underpin the most important aspects of human language.
The data that LLMs rely upon has a fundamental problem: it is entirely linguistic. All LMs receive are streams of symbols detached from their referents, and all they can do is find predictive patterns in those streams. But critically, understanding language requires having a grasp of the situation in the external world, representing other agents with their emotions and motivations, and connecting all of these factors to syntactic structures and semantic terms. Since LLMs rely solely on text data that is not grounded in any external or extra-linguistic representation, the models are stuck within the system of language, and thus cannot understand it. This is the symbol grounding problem: with access to just formal symbol system, one cannot figure out what these symbols are connected to outside the system (Harnad, 1990). Syntax alone is not enough to infer semantics. Training on just the form of language can allow LLMs to leverage artifacts in the data, but “cannot in principle lead to the learning of meaning” (Bender & Koller, 2020). Without any extralinguistic grounding, LLMs will inevitably misuse words, fail to pick up communicative intents, and misunderstand language.
I fucking loved windows 8, if I could I would still have the all screen start menu bastard.